IRRODL: Increasing access to Higher Education:
A study of the diffusion of online teaching among 913 college faculty
July - 2005
Increasing access to Higher Education:
A study of the diffusion of online teaching among 913 college faculty
Peter Shea
University at Albany, State University of New York
Alexandra Pickett
SUNY System Administration
Chun Sau Li
University at Albany, State University of New York
Abstract
Online learning environments provide an unprecedented opportunity to increase student access to higher education. Accomplishing this much needed goal requires the active participation and cooperation of university faculty from a broad spectrum of institutional settings. Although online learning has seen rapid growth in recent years, it remains a relatively small percentage of the entire curriculum of higher education today. As a relatively recent development, online teaching can be viewed through the lens of diffusion of innovation research. This paper reports on research from 913 professors from community colleges, four-year colleges, and university centers in an attempt to determine potential barriers to the continued growth in adoption of online teaching in higher education. It is concluded through factor and regression analysis that four variables are significantly associated with faculty satisfaction and their likelihood, therefore, to adopt or continue online teaching – these include levels of interaction in their online course, technical support, a positive learning experience in developing and teaching the course, and the discipline area in which they taught. Recommendations for institutional policy, faculty development, and further research are included.
Keywords: online teaching, faculty satisfaction, faculty development, diffusion of innovation, access, higher education, study
Online learning in higher education is a topic that has received much attention in recent years, in large measure due to its explosive growth. According to the Sloan-Consortium report, Sizing the Opportunity: The Quality and Extent of Online Education in the United States, it is estimated that more than 1.9 million college students were engaged in learning at a distance via Internet-based technologies in Fall 2003 and that this number is expected to grow to 2.6 million in Fall 2004. The authors also report that more than 33 percent of such students took all of their courses online, and more than 80 percent of US colleges now offer at least one fully online or blended course (Allen and Seaman, 2004). Others have reported similar growth rates for online education in the U.S. and Canada (Lewis, Levin, and Green, 1999; LaGrange and Foulkes, 2004; U.S. Department of Education, 2004). This growth is also reflected in the online program studied here, The State University of New York Learning Network (SLN), which in the 2003-2004 academic year offered more than 80 complete online degree programs to approximately 70,000 students enrolled across 40 campuses. These numbers compare to just eight online courses offered to 56 students in four institutions in the 1995-96 academic year.
The benefits cited by faculty of offering online learning opportunities to students have been well documented (Dziuban, Shea, and Arbaugh, 2005) and include greater and higher quality interaction with students (Kashy, Thoennessen, Albertelli, and Tsai, 2000; Hartman, Dzuiban, and Moskal 2000; NEA, 2001; Shea, Fredericksen, Pickett, Pelz, and Swan, 2001; Smith, 2001; Swan, Shea, Fredericksen, Pickett, Pelz, and Maher, 2000); increased convenience and flexibility for their teaching and students’ learning (Arbaugh, 2000; Hartman and Truman-Davis 2001; NEA, 2001); better access to student populations and increased access for students to higher education (NEA, 2001); enhanced knowledge of educational technology (Fredericksen, Pickett, Pelz, and Swan, and Shea, 2000; Rockwell, Schauer, Fritz, and Marx, 1999; Thompson, 2001), increased opportunities for professional recognition and research (Hartman and Truman-Davis, 2001; Hislop and Atwood, 2000; Smith, 2001), high levels of student learning (Hartman, Dzuiban, and Moskal 2000; NEA 2000, Shea et. al., 2001, 2002; Thompson, 2001), greater necessity and opportunity for more systematic design of online instruction and a corollary positive impact on student learning and on classroom teaching (Shea, Pelz, Fredricksen, and Pickett, 2003).
While these benefits suggest that most faculty members may be quite willing to engage in online teaching, experience indicates there are still significant barriers and resistance to such technology-mediated instruction. Commonly cited barriers include more time required (Clay, 1999; Hartman and Truman-Davis, 2001; Hislop and Atwood, 2000; NEA, 2001; Thompson, 2001; Schifter, 2000); inadequate compensation (Betts, 1998; NEA, 2001; Rockwell et al., 1999; Smith, 2001; Wolcott, 1997), ownership issues (NEA, 2001; Twigg, 2000; Werry and Mowbray, 2001); more work to develop and teach online (though possibly counterproductive to professional advancement) (Betts, 1998; Schifter, 2000), technical difficulties (Chizmar and Williams, 2001; NEA, 2001, Schifter, 2000), and inadequate training, support and the addition of new roles (e.g., faculty become the helpdesk) (Fredericksen et. al, 2000; Hartman and Truman-Davis, 2001; Schifter, 2000; Thompson, 2001).
If the benefits associated with online teaching are to be realized – especially those most clearly revered, such as increasing access to higher education – faculty participation and engagement is critical. Higher education enrollments are growing and are expected to continue to grow – for example between 1997 and 2003 an additional 111,225 students participated in higher education in New York State alone (The Nelson A. Rockefeller Institute of Government, 2004). While there has been a tremendous growth in the complete online learning enterprise, it still remains a small fraction of the higher-education curriculum relative to the traditional classroom – for example the Pew Internet and American Life Project estimates that, despite rapid growth in online learning, less than one percent of Americans who log onto the internet on a typical day do so to study online for college credit (Pew Internet and American Life Project, 2004). It is estimated that less than 10 percent of the curriculum of American colleges is available online (Mayadas, 2004), a trend that is also true within the organization studied here. While growth in online education has been dramatic, it seems clear that much more can be done to accommodate the increasing demand for higher education through online teaching and learning environments.
Part of the explanation for the limited use of online teaching and learning is its incompatibility with the teaching styles of many professors. It is often claimed that faculty are more likely to adopt Web-enhanced and hybrid options, rather than complete online teaching and learning (due in part to the complexity and time investments of the latter). While this makes sense intuitively – and despite the fact that more than 80 percent of public four-year colleges provide faculty access to course management systems to offer online learning – it is estimated that faculty only use them in 20 percent of their courses (Lynch, 2002). Thus, it appears that even Web-enhanced and hybrid uses of Internet-based technologies for higher education teaching and learning remain quite limited. Understanding and responding to the concerns of professors is crucial to the further expansion of online teaching and learning opportunities. In order to respond to bold calls for increasing the number of online courses and students by ten-fold in the next ten years (Mayadas, 2004), careful attention must be paid to the participation of such faculty, without whom even existing levels of online offerings will not be sustainable.
This study reports result of research on faculty satisfaction with online teaching conducted through a large, state-wide online program – the SUNY Learning Network. The SUNY Learning Network (SLN) represents fertile ground for investigations of faculty adoption of this innovation – more than 1,000 professors across a broad range of colleges teach using the technologies and supports provided through the program each semester. With the assistance of the SLN instructional design and technology support staff these faculty have developed, and the program has delivered more than 3,000 online courses to more than 250,000 student enrollments since 1996.
The issues surrounding faculty engagement and satisfaction in online teaching and learning have been explored by a variety of researchers. Faculty adoption and use of online learning technologies may be considered an instance of the larger realm of diffusion of innovation in higher education generally. A number of theoretical models on diffusion of innovation exist (Dooley, 1999; Hall, Wallace, and Dossett, 1973; Rogers, 1963, 2003) which are relevant to discussions of faculty engagement with online teaching. The most commonly cited model is Rogers’ (2003) who suggests that faculty go through several stages in the adoption process, which is influenced by specific characteristic of the innovation. The stages of adoption include knowledge of the innovation, persuasion, decision, implementation, confirmation, and in some instance, reinvention (Figure 1).
Figure 1. Rogers’ (2003) Diffusion of Innovation Model
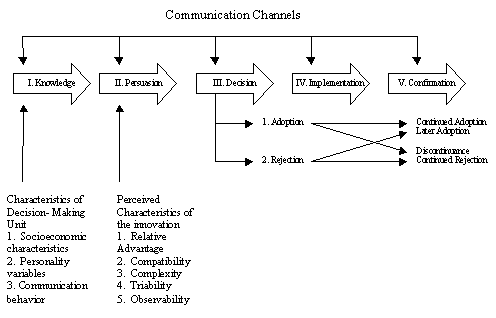
Within the knowledge stage, individual characteristics of the decision maker bear on whether the process will continue to the next stages – these include socio-economic and personality variables and communication behaviors (Rogers, 2003). Individual characteristics of the decision-maker support or undermine the decision to be persuaded in the next stage. The knowledge stage also has a bearing on administrative decisions about whom to consider for support or inclusion in online teaching initiatives. A good alignment of the appropriate individual characteristics should be assessed, because innovations tend to fail due to the audience to whom they are initially disseminated.
In the persuasion stage the individual considers the relative advantages, compatibility, “observability,” “triability,” and complexity of an innovation. Relative advantage refers to the degree to which the adopter perceives the innovation to represent an improvement in either efficiency or effectiveness in comparison to existing methods. The adoption of an innovation such as online teaching is, to a certain extent, contingent upon the existence and success of faculty development and training efforts. In these efforts it is essential that potential adopters are made aware of the relative advantages of the innovation under consideration. The online program studied here has made significant efforts in this regard; with more than 100 days of face-to-face training offered each year, the program has endeavored to ensure that new online professors are given relevant information on the advantages of online instruction. The relative advantages of online instruction communicated to new faculty include its flexibility, interactivity, and the programmatic and technical support offered by the SLN to students and instructors (Shea et. al., 2002).
Observability refers to the ease with which the technology can be seen, imagined, or described to the potential adopter (Rogers, 2003). Through the SLN’s faculty development process, new faculty members are provided access to views and examples of the technology and pedagogy of online learning. This is accomplished through access to both online courses for observation and an online all-faculty conference that allow new faculty to see the environment in which online teaching and learning occur. Through demonstration activities, potential adopters are assisted through this stage (Shea, Fredericksen, Pickett, and Pelz, 2004).
Triability refers to the capacity to experiment with the new technology before adoption. The greater the opportunity to test the new technology, the more likely it will be adopted (Rogers, 2003). Again, through the SLN’s all-faculty conference, through links to live and archived online courses for observation and through the provision of technical scaffolding, new faculty in the SLN are given ample opportunity to test online teaching before they actually engage in it. The all-faculty conference allows new instructors to engage in online teaching and learning in the same environment that their future students will use (Shea, Fredericksen, Pickett, and Pelz, 2004).
The fourth characteristic in Rogers’ model is complexity – the degree to which the innovation is difficult to understand or apply (Rogers, 2003). Managing complexity is among the greatest challenges to the diffusion of innovation. The online program studied here provides both technical and human resource support to assist faculty to deal with complexity issues. The provision of wizard-driven online course templates for the SLN’s course developers allows potential adopters to manage the complexity of creating a complete online course “from scratch.” With the click of a button, new faculty are prompted through the creation of an outline for their online course, which includes options for a course syllabus, a schedule, and learning modules with embedded documents for modules overviews, lectures, readings, assignments, tests and self tests, discussions, and small groups. Through this kind of scaffolding, new faculty are assisted to deal with the inherent complexity of designing a complete online course (Shea, Fredericksen, Pickett, and Pelz, 2004).
Beyond the technical assistance provided by scaffolding technologies as described above, the SLN provides programmatic support through its Multimedia Instructional Design (MID) group and faculty and student helpdesk (Shea, Pickett, and Pelz, 2004). The MID program consists of a core group of instructional designers and more than 40 campus-based instructional support professionals. The SLN provides training and community development infrastructure to create and sustain a culture across the system that supports faculty efforts to use technology effectively. Through MID training activities, an annual “summit,” and ongoing monthly meetings, the MID group shares and continues to grow the knowledge needed to support faculty’s decision to adopt and implement online learning. The SLN provides more than 100 days of training per year in the design, development, delivery, and assessment of online teaching and learning.
The SLN’s faculty and student helpdesk provide a single point of contact to address technical issues as they arise in the development and delivery of online learning. To obtain rapid assistance with technical issues, faculty may contact the SLN’s helpdesk via phone, email, or via Web-based form. Providing such support reduces the threat that the complexity of the technology will impede adoption or lead to cessation of use of innovations such as online teaching. The SLN’s student helpdesk removes the burden from faculty of handling student technical support issues, another threat that exists between the decision and confirmation stages in the Roger’s diffusion model.
In order to assess the functioning of the program, each semester the program
implements an online survey of both faculty and students. The faculty survey
attempts to collect various measure of satisfaction and solicits faculty reactions
to different components of the online teaching experience. A copy of the questionnaire
is included in appendix A. This survey provides opportunity to assess certain
elements of Rogers’ diffusion of innovation theory – particularly
the decision and confirmation stages of the model, and to determine whether
and how the model applies to the issue of faculty adoption of online teaching
within the broad context represented by the SLN. The measures of satisfaction
that the survey items solicit are measures of confirmation in the model. Lack
of satisfaction or confirmation assessed through the survey may point to factors
in the decision stage that explain likelihood of continuation or discontinuation.
Responses to items may provide alternative rationale that support, or are not
accounted for, by the model. Additionally, given the relatively large sample
size and number and diversity of institutions represented, other organizations
involved in the development of online learning initiatives may be interested
in these results for lessons learned and potential obstacles to avoid in diffusing
technological innovations to higher education faculty.
Method
Participants
Participants in this study included 913 faculty members who taught at 33 colleges in the SLN in the 2003-2004 academic terms. Approximately 43 percent of the respondents were male and 57 percent were female. The age range included faculty who were under age 25 (less than .2 percent) to over age 66 (more than 5 percent). The largest group was age 46-55 (nearly 40 percent) followed by 56-65 (30 percent). Additional demographic characteristics of faculty respondents are summarized in Table 1.
Table 1. Demographic Characteristics of Faculty Respondents
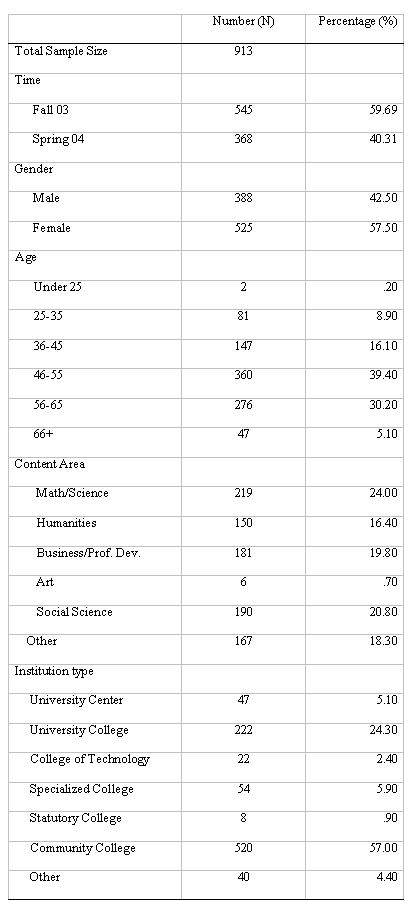
This sample represents approximately 34 percent return rate for these semesters. This rate of response, while low, is in alignment with rates reported by others using online survey methods (Sheehan, 2001). It is hypothesized that many Internet users are “survey saturated,” and inasmuch as assessments are implemented each semester in this program, faculty in the online environment studied here may also suffer from such overload – leading to lower response rates. Given the nature of the sample, which was limited and self selected, caution needs to be taken in applying these results – though this is a relatively large and diverse sample, there may still be issues of generalizability to the larger population. The levels of satisfaction presented here may be a function of the sample, again, though large and diverse, members of the sample may be more interested or simply more persistent and diligent and thus could be more satisfied than non-respondents. It must be admitted that inter-institutional research on recent technological innovations, such as presented here, does present certain challenges; for example, comparable demographic information for individual online faculty across these institutions is not collected or maintained in any single database, making estimates of generalizability to broader population parameters difficult to derive.
Instrument
Participants in the study responded to a 35 item survey assessing their levels of satisfaction, interaction, technical preparedness, technical difficulties, time investment, appropriateness of the online environment (for their discipline), student learning, and the influence of the online course development and delivery experience on their understanding of new methods of pedagogy, assessment, and its likely impact on their classroom teaching. Most items included were composed using a five-point Likert-type scale in which participants responded to statements about their online teaching experience. A copy of the instrument is included in Appendix A.
Procedure
Faculty were contacted via email three times during an eight-week period asking for their participation in both the fall 2003 and spring 2004 academic semesters. Respondents were also solicited through posted announcements on the SLN’s website. To encourage faculty participation, local campus support groups were also contacted by the researchers. The survey was available in an online format, and faculty were prompted to complete the survey when they logged into the online teaching and learning system. Instructors completed the survey using a Web-based form. The survey was also accessible from a link sent to the faculty in their email.
Faculty from 36 of the 40 campuses offering courses (90 percent) were represented.
These campuses were fairly representative of the overall categories of colleges
eligible to participate. Those campuses that are not represented included two
community colleges, one four-year college, and one university center –
i.e., campuses that were not represented did not cluster around a single institution
type.
Results
Descriptive results
Generally speaking, results for the survey disclose that respondents were highly satisfied with the experience of developing and teaching online courses. Approximately 90 percent reported that they were satisfied with the course they had just completed, with online teaching in general, and that their students learned a great deal in their online course (see Table 2). A large majority of the faculty reported they felt the online environment was appropriate for teaching in their content area (93.0 percent), and that they would like to teach an online course again (97.6 percent), or would recommend online teaching to a colleague (91.9 percent) (see Table 3). When asked to compare the performance of students in the online course they just finished teaching with the performance of students in similar courses they had taught in the classroom, the majority felt there was no difference, although approximately 33 percent of respondents thought that their online students performed better than classroom-based students, and only about 9 percent felt that their classroom students performed better (see Table 4).
Table 2. General faculty satisfaction with online teaching (N=913)
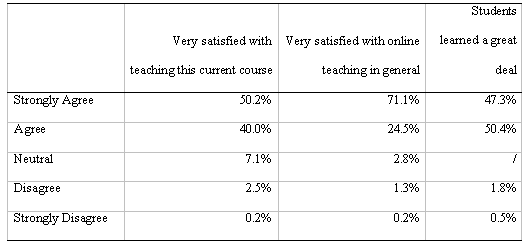
Table 3. Additional indicators of faculty satisfaction with online teaching (N=913)

Table 4. Faculty perception of online and classroom students’ performance (N=913)

Factor analysis
To determine which sets of variables in the overall results might form coherent subsets, a factor analysis was performed on the data. Variables that correlate with each other, but are, for the most part, independent of other subsets are grouped into factors. Such factors are thought to reflect underlying processes that are represented by the correlations between the variables (Tabachnick and Fidell, 2001). Analysis of the results via factor analysis may provide guidance in determining the validity of the Rogers model in a number of ways, for example do the results cohere in ways that reflect stages in the model and the overall results?
In the factor analysis questions 5 to 33 were input to examine their correlations. It was found that questions 5, 7, 8, 16, 26, and 28 had small correlation coefficients (less than .30) so that they were not included in the analysis following conventions established for this statistical approach (Tabachnick and Fidell, 2001). The correlation matrix of the remaining 21 items from the faculty online teaching and learning survey is presented in Table 5. A principal component extraction method with oblique rotation was performed to determine the dimensionality of the 21 items. Delta was equal to zero and Bartlett’s test of sphericity was acceptable for factor analysis (c2 = 4523.80, p<.001). Five factors were extracted and 57.2 percent of total variance could be accounted for. Variables are ordered and grouped by size of their loading to facilitate interpretation. The pattern matrix is presented in Table 6; the five factors were labeled as: Interaction, Time, Faculty Learning, Technical Support, and Satisfaction Measures.
Reliability analysis was performed to examine the internal consistency of the factors. Results showed that Interaction, Faculty Learning, and Satisfaction Measures have satisfactory internal consistencies, the Cronbach’s alphas were .81, .78, and .75, respectively. The internal consistencies of Time and Technical support were moderate, with coefficients of .57 and .60.
Table 5. Item Correlation Matrix
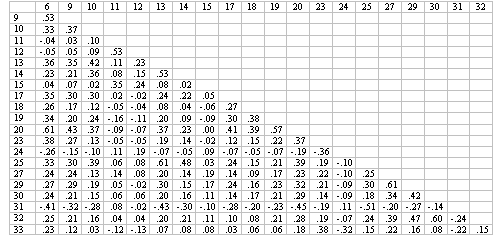
Table 6. Pattern Matrix

Descriptive analysis
The factor values were obtained by calculating the mean of the items within the factor. Table 7 presents mean score, standard deviation and Pearson correlation coefficients of the factors: Interaction, Time, Faculty Learning, Technical Support, and Satisfaction Measures. It was found that faculty satisfaction was significantly and positively associated with interaction ( =.45, p<.001), faculty learning ( =.38, p<.001), and technical support ( =.33, p<.001).
Table 7. Mean, Standard Deviation, Correlations
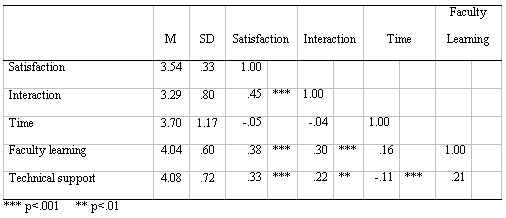
Based on previous investigations the model which emerges from the factor analysis appears to reveal underlying variables important to faculty satisfaction and the likelihood to adopt or continue to engage in online teaching. As noted in the review of literature above, each of these clusters of variable reflect important considerations identified by previous investigators analyzing faculty engagement with online teaching. Some researchers (e.g., Nachmias, 2002) have tied these variables to a diffusion model such as Roger’s, and they do appear to fit well into one or more of the stages he outlines – each factor represents a well defined and recognized support or impingement on satisfaction with the experience of developing and teaching in an online environment and subsequent decisions to reject, adopt, or persist with it. One test of these categories is the degree to which the satisfaction variables are associated with the others – time, technical support, faculty learning, and interaction variables – identified in the factor analysis.
To confirm the relative usefulness of these factors in understanding faculty engagement and persistence with online teaching, a regression analysis was conducted. The results are presented below.
Multiple regression analysis
A sequential multiple regression was applied to examine the relationship between online teaching satisfaction and other variables. The dependent variable was online teaching satisfaction as identified by the cluster of variables in the factor analysis, the independent variables were Time, Technical Support, Faculty Learning, Interaction, demographic (such as gender, age) and program information (such as content, institution type, and etc.), and reason of online teaching.
Results of the assumption check led to transformation of the variables to reduce skewness and number of outliers, and to improve the normality, linearity, and homoscedasticity of the residuals. Logarithmic transformations were used on the Time, Faculty Learning, Technical Support, and Satisfaction Measures. The Interaction factor was not transformed because the scores of skewness and kurtosis were small, approximately equal to zero. Additionally, with the use of a p<.001 criterion for Mahalanobis distance, 15 cases were identified as outliers and regarded as missing data.
The intercept, the un-standardized ( ) and standardized regression coefficients ( ), R2 statistics, adjusted R2, and change in R are presented in Table 8. R was significantly different from zero at the end of each step, after Step 5, with all IVs in the equation, R=.59, F(33, 864)=14.30, p<.001. After Step 1, the regression of the Interaction factor on Online Teaching Satisfaction factor resulted in adjusted R2 = .22, Finc(1, 896) =258.62, p<.001. After Step 2, with the Technical Support factor added to the prediction of Online Teaching Satisfaction, R2 = .29, Finc(1, 895) =76.17, p<.001. The R2 change was again significant. The factors for Faculty learning and Time were added into the equation in Steps 3 and 4 with adjusted R2 = .31, Finc (1, 894) =33.43, p<.001, and adjusted R2 = .31, Finc (1, 893) =.49, p>.05, respectively. The R2 change was found to be significant in Step 3, but not significant in Step 4. After Step 5, the variables of instructor gender, age, content area taught, institution type (community college through university center), numbers of course enrollments, years of online teaching experience, primary reason for online teaching, and the extent to which the course was fully developed before it began were added into the equation, the adjusted R2 = .33, Finc (1, 864) =1.95, p<.01. The regression analysis showed that Interaction, Technical Support, Faculty Learning significantly contribute to predict the satisfaction of faculty teaching, whereas the time variables did not. The content area variable was significant and implies that instructors of math/science, humanities, business/professional development tend to report higher levels of satisfaction than instructors in other discipline areas. The other demographic and contextual variables were not significant.
Table 8. Sequential Regression on Faculty Online Teaching Satisfaction

Finally, we performed a regression analysis on those significant factors and results showed that R was .57 and adjusted R2 was .32, which indicated 32 percent of total variance in faculty online teaching satisfaction could be accounted for by measure of interaction, technical support, faculty learning, and discipline area of instruction - math/science, humanities and business/professional tend to express higher levels of satisfaction. Presented in Table 9 are the un-standardized, standard error, and standardized regression coefficients. Examination of the tests revealed that online teaching satisfaction was linearly related to interaction (t=12.76, p<.001), technical support (t=7.94, p<.001), faculty learning (t=5.52, p<.001), and discipline area of instruction - Math/Science (t=4.05, p<.001), Humanities (t=3.04, p<.01), and Business/Professional (t=2.17, p<.05).
Table 9. Contributions of Predictors to Faculty Online Teaching Satisfaction
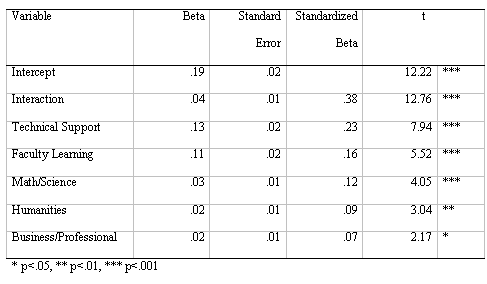
Discussion
The results presented here suggest that interaction, technical support, opportunities for learning, and discipline-specific factors are significantly linked with faculty satisfaction in online teaching, including measures that indicate adoption and continued engagement with this innovation – two elements of the Rogers diffusion of innovation model. We use the terminology “suggests” intentionally – it appears from these results that faculty satisfaction in online teaching may also depend on factors not identified here, as a significant proportion of the variance in the factor reflecting satisfaction remains unexplained by this study. The results reported here, however, are consistent with concerns frequently cited in the literature on faculty engagement in technology-mediated instruction. Each of the variables is discussed below.
Interaction
Perhaps the most frequently cited variable in discussions of quality in online
learning environments is interaction. As indicated above, high levels of interaction
are frequently mentioned as one of the potentially positive aspects of online
teaching and learning. Enhanced opportunities for interaction online, when properly
managed so as not to be overwhelming, may be reasonably associated with the
relative advantage in the Roger’s diffusion of innovation model. The results
presented here support the centrality of well-managed interaction and suggest
that levels of interaction influence faculty decisions to adopt, reject, or
continue with online teaching. The factor reflecting interaction in this study
included faculty perceptions of levels of interaction with their students, between
their students, their level of knowledge of students, and faculty feelings of
isolation in the online environment. The multivariate regression analysis indicates
that higher reported levels of interaction with and between students, greater
knowledge of students, and lower feelings of isolation are positively associated
with the variables in the satisfaction factor – including reported likelihood
to teach again online, likelihood to recommend a colleague to teach online,
satisfaction with online teaching in general, and satisfaction with the specific
online course just completed. Each of these measures reflects components of
the diffusion model proposed by Rogers (2004). For example, interaction in online
environments can represent a measure of complexity in the model – which
may impinge upon adoption and continued used. Faculty members new to online
teaching, often report being overwhelmed by increased interaction levels. The
supports offered to instructors in this program (Shea, Fredericksen, Pickett,
and Pelz, 2004) are designed, in part, to allow them to cope with higher levels
of interaction and thus overcome this complexity issue reflected in the Roger’s
model. The results reported here suggest that faculty who were likely to continue
to engage in this innovation as measured by the satisfaction factor, were able
to view interaction in a positive light rather than as overwhelming.
Technical support
As with the interaction variables, the same may be said about the significance
of faculty satisfaction with technical supports and technical barriers –
the addition of this variable to the regression resulted in a significant change
in the adjusted R2 value. Positive perceptions regarding the usability of the
SLN’s Learning Management System, low levels of reported technical difficulties,
and higher levels of satisfaction with faculty support services, contribute
significantly to the regression equation for the cluster of satisfaction variables
previously identified. The existence of technical support may also reasonably
be associated with overcoming complexity issues identified in the Roger’s
model. It appears that faculty who reported satisfaction with these elements
of the technical and programmatic support system, were more satisfied as measured
by the factor containing items that reflect satisfaction with online teaching
and the course they just taught, likelihood to teach online again, likelihood
to recommend a colleague, etc.
Faculty learning
The variables included in this factor focused around the opportunities for
gaining new knowledge, skills, and insights about the act of teaching as a result
of the experience of developing and instructing an online course. Faculty who
were more likely to report that this experience afforded them opportunities
to consider – alternative means of instruction, alternative means of assessment,
how they taught in the classroom, and that developing and teaching and online
course would likely improve how they taught in the classroom – accounted
for more of the variance in responses to the cluster of satisfaction variables.
Faculty who felt that developing and teaching online was a learning experience
for themselves, tended to report more positive outcomes – higher levels
of satisfaction, greater likelihood to teach again, greater likelihood to recommend
a colleague, etc., important considerations for the diffusion of this innovation.
Support provided to faculty in the training cycle affords opportunities for
reflecting on developing and teaching online courses as a learning experience
(Shea, Fredericksen, Pickett, and Pelz, 2004); such an orientation may be viewed
as an additional “relative advantage” in Roger’s model for
these instructors when considering whether to adopt or continue to use this
innovation. It appears that this advantage is associated with satisfaction with
this innovation and increased probability of adoption or continuation.
Time
Concerns about the additional time and effort associated with online teaching
are quite common in the literature surrounding e-learning (Schifter, 2000; Betts,
1998; Clark 1993; Taylor, 1991). The variables included in the factor associated
with time in this study did not contribute significantly to the regression equation.
One interesting correlation may help explain this result. While concerns about
additional time requirements for online teaching would suggest that faculty
who reported high levels of time commitment to develop and deliver online courses
would be more likely to express dissatisfaction, the opposite appears to be
true. Faculty who reported more time in developing their online course reported
more satisfaction with the course they had just finished teaching. Other correlations
concerning time appear to be inconclusive or non-significant – for example,
combining the means of all the satisfaction measures and comparing satisfaction
levels by reported teaching time and reported course development time reveals
no significant differences (i.e., faculty who reported that the spent only 30
hours developing their course were no more or less satisfied than those who
reported spending in excess of 150 hours). While few researchers have engaged
in comparisons measuring actual time investment in online and traditional teaching,
the results of this line of inquiry suggest that they may actually be equivalent
(e.g., Lazarus, 2003), thus leading to the conclusion that, in the end, these
time considerations do not significantly influence levels of satisfaction with
online teaching. Finally, it is not unlikely that this factor needs to be refined
to increase reliability; additional items related to time should be generated
and included in an expanded and more reliable factor.
Discipline specific variables
The evidence suggests a small but significant effect for the discipline of
instruction on the factors reflecting faculty satisfaction. It appears that
faculty identifying themselves as teaching courses in faculty in Math/ Science,
Humanities and Business/ Professional Development have higher levels of satisfaction
than those identifying themselves as teaching in the Social Sciences, Art, or
“Other” categories. Given the relatively small contribution of this
factor and the skewness of the members within each of the categories (e.g.,
only nine faculty reported teaching within the “Art” discipline
area), this finding requires additional investigation and confirmation.
Recommendations
These results suggest that a number of variables significantly correlate with
faculty satisfaction in online teaching. In order to meet the challenge of increasing
access to higher education through the diffusion of online teaching and learning,
institutions need to be mindful of the potential to impact faculty in at least
three large, inter-related areas: Faculty Development, Technical Support, and
Course Design. These are discussed further below.
Faculty development
It is now commonly accepted that high quality online teaching and learning
must be supported through systematic and well organized faculty development
initiatives. In previous research we have outlined the nature of the faculty
development process in the SLN program studied here (Shea et al. 2001, 2002,
2004) and presented results indicating that faculty development efforts influence
not only faculty satisfaction, but impact on the reported learning and satisfaction
of the students of faculty who receive such training (Shea, Pickett, and Pelz,
2004). The current study also implies that faculty development efforts that
encourage instructors to participate in ways that highlight the opportunity
to explore, learn, create, and apply their learning to their traditional teaching
methods, may lead to higher levels of adoption and continued use. Engaging experienced
online faculty in training and development efforts who can attest to this impact,
are likely to strike a resonant chord with other potential adopters of this
innovation. Veteran faculty members played a large role in training efforts
in this program – experienced online instructors are invited to new faculty
training sessions and their experiences, both positive and negative, allow the
uninitiated to better understand the nature of this innovation, thus increasing
opportunities deemed facilitative in Roger’s diffusion model (more on
this below). Planning for substantial online teaching and learning programs
should include adequate resources allocated to faculty development and support
(including policies recognizing online teaching in tenure and promotion considerations).
The program studied here allocates an estimated 5-6 month development period
for the design, development, and implementation of each cohort of faculty-created
online courses. Instructional designers and trainers spend 100 days per year
in face-to-face training and many additional hours involved in follow-up, online
support. While resource intensive, such efforts play a crucial role in assisting
faculty through the persuasion and decision stages of the technology adoption
model posed by Rogers. For example, learning from peers who have already adopted
the innovation has an impact on at least three of the five elements of the persuasion
stage in Roger’s model, including relative advantage, compatibility, and
complexity. Understanding relative advantage is made easier when a successful
adopter explains it to a new online instructor – real and credible examples
can be offered, as is the case in trainings offered in this program. Learning
from veteran online faculty peers also demonstrates that online teaching is,
at least potentially, compatible with academic culture and values – if
it were not peers would not make a positive adoption decision. Opportunity to
interact and learn with experienced online instructors also provides new faculty
with strategies that have been successfully employed to overcome complexity
issues – which are highlighted in the trainings offered in this program
(Shea, Fredericksen, Pickett, and Pelz, 2004).
Technical support
Technical support is also recognized as a crucial element in the success of
significant online teaching and learning endeavors. The current study supports
this largely anecdotal conclusion with result from nearly 1,000 online instructors.
Organizations that wish to scale-up to meet the growing demand for quality and
access to online higher education have recognized the need for technical support
for students – these findings suggest the same level of attention may
be needed to encourage ongoing faculty adoption and continued engagement with
online teaching. The continued diffusion of this innovation may rest upon the
ability to persuade faculty that adequate technical support will be available
as they decide whether to participate. Planning for significant online teaching
and learning programs should include adequate resources allocated to technical
support. The program studied here includes a professionally staffed helpdesk
that is shared by the 40 colleges participating in the program. Such resource
allocation avoids un-needed redundancy and avoids costs, while building a shared
knowledge base that benefits all. In the program studied here, technology supports
are designed in ways that assist faculty with other two elements of the persuasion
stage of Roger’s model not mentioned above: “triability” and
“observability.” For example, through the “All Faculty Conference”
the program offers new faculty an opportunity to experience online learning
as their students will, in the same online environment in which they will teach.
Opportunities to engage in the technology environment (and to simultaneously
gain exposure to online pedagogy) increase the “triability” and
“observability” of the online teaching innovation, reduces the mystery,
and based on these results, may reasonably be expected to increase the likelihood
of adoption and continuation. Institutions wishing to increase access to their
academic offerings through online teaching and learning may benefit from offering
similar opportunities to address these elements of the diffusion of innovation
challenge.
Interaction
Again, while it is commonly understood that high levels of interaction in online
higher education is associated with student reports of satisfaction and learning,
these results suggest the same is true for faculty. Given the results relative
to the issue of interaction several recommendations seem reasonable. Large scale
implementations of online teaching and learning often require the use of scaffolding
mechanisms to assist faculty with issues of complexity identified in the Roger’s
model – in the case of the SLN studied here, these include the use of
course templates that encourage sound instructional design and organization,
the facilitation of productive discourse, and effective direct instruction (Shea,
Fredericksen, Pickett, and Pelz, 2004). Common, yet flexible course designs
that enable instructors to encourage high levels of interaction with and between
students coupled with faculty development efforts that support their implementation
are likely to increase interaction and faculty satisfaction as well. Ongoing
support from and access to academic technology specialists and or instructional
designers has allowed the program studied here to grow and succeed. The expense
for these human resource supports may be shared between institutions to avoid
costs, but the provision for such supports seems integral to the successful
adoption of this innovation.
It has been argued here that online teaching and learning represent an enormous opportunity to increase access to higher education – a much needed and unqualified benefit in our growing knowledge-based society. While online learning remains a relatively small segment of the entire higher education curriculum, better understanding of how this innovation may be successfully diffused to a wider audience of faculty, will enable institutions to grow to meet a larger portion of the demand for higher education in the years ahead. It is clear that limitations in this study call for additional research to understand faculty adoption and continued use of this innovation; there is much we do not know about why faculty accept or reject online teaching. It also appears clear that attention paid to the concerns of professors will facilitate their acceptance of online teaching, and evidence presented here provides some guidance in this regard. Appropriate emphasis on interactive pedagogy, faculty training, technical support, and recognition of the time-investment needed to develop and deliver online instruction will advantage institutions that are wishing to increase access to their instructional programs, and will help ensure they are delivered in a high quality online format.
Acknowledgement
The authors wish to thanks the Office of SUNY Learning Environments for its direct support of this research. The authors also wish to express their gratitude to the Director of the SUNY Learning Network and the SLN Team – especially Doug Cohen for his outstanding technical support of this research. SUNY Learning Environments is an office of the Provost and Vice Chancellor of the State University of New York.
References
Allen, E. I., and Seaman, J. (2004). Entering the Mainstream:
The quality and extent of online education in the United State, 2003 and 2004.
Needham, MA.: Sloan Center for Online Learning in Education (SCOLE).
Arbaugh, J. B. (2000). Virtual classroom characteristics and student
satisfaction in internet-based MBA courses. Journal of Management Education
24, 32 – 54.
Betts, K. S. Factors influencing faculty participation in distance education in postsecondary education in the United States: An institutional study. (Doctoral dissertation. The George Washington University, 1998). Dissertation Abstracts International: UMI.
Chizmar, J. F., and Williams, D. B. (2001). “What Do Faculty
Want?” EDUCAUSE Quarterly 24(1), 18 – 24.
Clark, T. (1993). Attitudes of Higher Education Faculty toward
Distance Education: A National Survey. The American Journal of Distance Education
7(2), 19-33.
Clay, M. (1999). Faculty attitudes toward distance education at
the State University of West Georgia. University of West Georgia Distance
Learning Report. Retrieved January 28, 2005 from: http://www.westga.edu/~distance/attitudes.html
Dooley, K. E. (1999). Towards a Holistic Model for the Diffusion
of Educational Technologies: An integrative review of the educational innovation
studies. Educational Technology and Society 2(4), 35 – 45.
Dziuban, C., Shea, P., and Arbaugh, J. (2005). Faculty roles and satisfaction in ALNs. In S. R. Hiltz and R. Goldman (Eds.) Learning together online: Research on asynchronous learning networks. (pp. 169-190). Mahway, NJ: Lawrence Elrbaum Associates.
Fredericksen, E., Pickett, A., Pelz, W., Swan, K., and Shea, P.
(2000). Factors influencing faculty satisfaction with asynchronous teaching
and learning in the SUNY Learning Nework, Journal of Asynchronous Learning
Networks 4(3), 245 – 278.
Hall, G. E., Wallace, R. C., and Dossett, W. A. (1973). A developmental conceptualization of the adoption process within educational institutions. Austin Research and Development Center for Teacher Education: University of Texas at Austin.
Hartman, J., Dzuiban, C., and Moskal, P. (2000). Faculty Satisfaction
in ALNs: A dependent or independent variable. Journal of Asynchronous Learning
Networks 4(3). Retrieved December 21, 2004 from: http://www.aln.org/alnweb/journal/jaln-vol4issue3.htm
Hartman, J. L., and Truman-Davis, B. (2001). Factors relating to
the satisfaction of faculty teaching online courses at the University of Central
Florida. In J. Bourne and J. C. Moore (Eds.) Online Education Volume 2: Learning
Effectiveness, Faculty Satisfaction, and Cost Effectiveness. Needham, MA.:
SCOLE.
Hislop, G., and Atwood, M. (2000). ALN teaching as routine faculty
workload. Journal of Asynchronous Learning Networks 4(3). Retrieved
December 21, 2004 from: http://www.aln.org/alnweb/journal/jaln-vol4issue3.htm
Kashy, E., Thoennessen, M., Albertelli, G., and Tsai, Y. (2000).
Implementing a large on-campus ALN: Faculty perspective. Journal of Asynchronous
Learning Networks 4(3), 231 – 244.
LaGrange, A., and Foulkes, E. (2004). Emergent framework for ICT integration within faculties of education in Canada. Ottawa: Report prepared for Industry Canada on behalf of Canadian Association of Deans of Education.
Lazarus, B. (2003). Teaching courses online: How much time does
it take? Journal of Asynchronous Learning Networks 7(3), 47 –
54.
Lewis, L., Levin, D., and Green, B. (1999). Distance education at postsecondary institutions: 1997-98. National Center for Education Statistics, U.S. Department of Education.
Lynch, D. (2002, January 18). Professors should embrace technology in courses. Chronicle of Higher Education Review Retrieved June 17, 2005 from: http://chronicle.com
Mayadas, F. (2004). Opening remarks. Presentation at the
Annual Meeting of the Sloan Asynchronous Learning Networks Conference in May
2004 at Orlando FL.
Nachmias, R. (2002). Research framework for the study of a campus-wide
web-based academic instruction project. Internet and Higher Education 5
(3), 213 – 229.
Nachmias, R. (2002). A research framework for the study of a campus-wide
Web-based academic instruction project. Internet and Higher Education
5(3), 213 – 229.
National Education Association (2000). A survey of traditional and distance learning higher education members. Washington DC.
National Education Association (2001). Focus on distance education.
Update 7(2). Washington, DC.
Pew Internet and American Life Project (2004). Daily Internet Activities. Retrieved January 26, 2005 from: http://www.pewinternet.org/trends/Daily_Activities_4.23.04.htm
Rockwell, K., Schauer, J., Fritz, S. M., and Marx, D. B. (1999).
Incentives and obstacles influencing higher education faculty and administrators
to teach. Online Journal of Distance Learning Administration 2(4). Retrieved
December 21, 2004 from: http://www.westga.edu/~distance/rockwell24.html
Rogers, E. M. (1963). Diffusions of Innovations. New York: Free Press. First Edition
Rogers, E. M. (2003). Diffusions of Innovations. New York: Free Press. Fifth Edition
Schifter, C. C. (2000). Faculty Participation in Asynchronous Learning Networks: A Case Study of Motivating and Inhibiting Factors, Journal of Asynchronous Learning 4(1), 15 – 22.
Shea, P., Fredericksen, E., Pickett, A., Pelz, W., and Swan, K.
(2001). Measures of Learning Effectiveness in the SUNY Learning Network. In
J. Bourne and J. C. Moore (Eds.), Online Education (Volume 2) Learning effectiveness,
faculty satisfaction, and cost effectiveness (p. 7-31). Needham,
MA.: SCOLE
Shea, P., Pelz, W., Fredericksen, E., and Pickett, A. (2002). Online
teaching as a catalyst for classroom-based instructional transformation. In
J. Bourne and J.C. Moore (Eds.) Elements of quality online education
(p. 103-126). Needham, MA.: SCOLE
Shea, P., Pickett, A., and Pelz, W. (2003). A follow-up study of
teaching presence in the online program. Journal of Asynchronous Learning
Networks 7(2), 61 – 80.
Shea, P., Pickett, A., and Pelz, W. (2004). Enhancing student satisfaction
through faculty development: The importance of teaching presence. In J. Bourne
and J.C. Moore (Eds), Elements of quality online education: Into the
mainstream - Volume 5 in the Sloan-C Series (p. 39-59). Needham, MA.: Sloan
Center for Online Education.
Shea, P., Fredericksen, E., Pickett, A., and Pelz, W. (2004). Faculty
development, student satisfaction, and reported learning in the SUNY Learning
Network. In T. Duffy and J. Kirkley (Eds.) Learner-centered theory and practice
in distance education (p. 343-377). Mahway, NJ.: Lawrence Elrbaum Associates.
Sheehan, K. (2001). Email survey response rates: A review. Journal
of Computer Mediated Communication 6(2). Retrieved May 12, 2005 from: http://jcmc.indiana.edu/vol6/issue2/sheehan.html
Smith, L. (2001). Faculty satisfaction in LEEP. A web-based graduate
degree program in library and information science. In Bourne and Moore
(Eds.) Online Education (Volume 2) Learning effectiveness, faculty satisfaction,
and cost effectiveness (p. 87-108). Needham, MA.: Sloan Center for Online
Education.
Swan, K., Shea, P., Fredericksen, E., Pickett, A., Pelz, W., and
Maher, G. (2000). Building Knowledge Building Communities: Consistency, contact
and communication in the virtual classroom. Journal of Educational Computing
Research 23(4), 359 – 383.
Tabachnick, B. G., and Fidell, L. S. (1996). Using multivariate statistics. (3rd. Ed.), New York: Harper Collins College Publishers.
Taylor, J. C., and White, V. J. (1991). Faculty attitudes towards
teaching in the distance education mode: An exploratory investigation. Research
in Distance Education 3(3), 7 – 11
The Nelson A. Rockefeller Institute of Government, State University of New York (2004). New York State Statistical Yearbook online. Retrieved December 21, 2004 from: http://www.nysstatistics.org/yearbook/04/data/J_1.pdf
Thompson, M. (2001). Faculty Satisfaction in Penn States World
Campus. In J. Bourne and J. C. Moore (Eds.) Online Education (Volume
2) Learning effectiveness, faculty satisfaction, and cost effectiveness,
(p. 129-144). Needham, MA.: Sloan Center for Online Education.
Twigg, C. (2001). Who Owns Online Courses and Course Materials? Intellectual Property Policies for a New Learning Environment. Retrieved December 21, 2004 from: http://www.center.rpi.edu/PewSym/mono2.html
U. S. Department of Education, National Center for Education Statistics
(2004). The Condition of Education 2004 (NCES 2004-077). Washington,
DC.: US Government Printing Office.
Werry, C., and Mowbray, M. (2001). Online Communities: Commerce
community action, and the virtual university. Upper Saddle River, NJ.: Prentice
Hall
Wolcott, L. L. (1997). Tenure, promotion, and distance education:
Examining the culture of faculty rewards. The American Journal of Distance
Education, 11(2), 3 – 18.
Appendix A
Online Faculty Survey Questions
1. Gender:
o Female
o Male
2. Age:
o Under 25
o 25-35
o 36-45
o 46-55
o 56-65
o Over 65
3. Content Area (If you answered "Other" to question 3, please specify
your content Area)
o Math/Science – includes Engineering-Technology-Health
o Humanities
o Business/ Professional Development
o Art
o Social Science
o Other
4. I taught this course through a (college or institution type)
o University Center
o University College
o Health Science Center
o College of Technology
o Specialized College
o Statutory College
o Community College
o Other
5. What was your level of personal computer skills before developing this online
course?
o High – Quite competent – experience with the Internet
o Average – Some knowledge of one or more applications
o Low – Had used a personal computer
o Zero – Had never used a personal computer
6. Overall I was very satisfied with teaching this online course.
o Strongly agree
o Agree
o Neutral
o Disagree
o Strongly disagree
7. How many students were in this course?
o 1-10
o 11-20
o 21-30
o 31-40
o Over 40
8. How many times have you taught this course online through the online program?
o This was my first time.
o This was my second time.
o This was my third time.
o Fourth or more.
9. Overall my students learned a great deal in this online course.
o Strongly agree
o Agree
o Disagree
o Strongly disagree
10. If you have ever taught this course in the classroom, how would you compare
your online students' performance to the performance of your classroom students?
o My online students performed better
o No difference
o My online students did not perform as well
o I have not taught this course in the classroom
11. Compared to the classroom, the preparation time for this online course
took
o Much more time
o More time
o About the same amount of time
o Less time
o Much less time
o I did not develop this course
o I do not teach in the classroom
12. Compared to the classroom, teaching this course took
o Much more time
o More time
o About the same amount of time
o Less time
o Much less time
o I do not teach in the classroom
13. Compared to classroom-based teaching, rate your level of interaction with
your online students
o Much higher
o Higher
o About the same
o Lower
o Much lower
? I do not teach in the classroom
14. Compared to classroom-based teaching, rate the level of interaction between
your online students
o Much higher
o Higher
o About the same
o Lower
o Much lower
o I do not teach in the classroom
15. How many hours would you estimate you spent developing your online course?
o 0-30
o 31-60
o 61-90
o 91-120
o 121-150
o More than 150
16. When you began teaching your online course, to what extent was your course
complete?
o 100%
o 75%
o 50%
o 25%
o Less than 25% complete
17. Do you think the online environment is appropriate for teaching your course
content?
o Yes
o Undecided
o No
18. Would you like to teach an online course again?
o Yes
o Undecided
o No
19. Would you recommend teaching online to a colleague?
o Yes
o Undecided
o No
20. How satisfied are you with online teaching in general?
o A great deal
o Somewhat
o Neutral
o Not very much
o Not at all
21. How did you come to develop this online course?
o I was asked to/required to
o I volunteered
22. Which of the following was your primary reason for teaching this course
online? If you answered "Other" to question 22, please specify your
primary reason for teaching this course online:
o Curiosity
o Marketability of the skills
o Wanting/ needing to telecommute
o Course is only offered online
o Interest in technology/Internet
o Research
o Fear of being left behind
o Interest in online teaching and learning
o Other
23. Overall, how satisfied are you with the online program support and services?
o A great deal
o Satisfied
o Neutral
o Not very satisfied
o Not at all
24. Do you feel technical difficulties made it more difficult to teach in this
environment than in the classroom?
o Not applicable – I did not have any technical difficulties
o Not applicable – I had technical difficulties but they did not
affect my teaching
o Not any more difficult than in the classroom
o Yes – some somewhat more difficult
o Yes – much more difficult
o I do not teach in the classroom
25. Compared to the classroom how well did you get to know your students online?
o Much better
o Better
o The same
o Not as well
o Not at all
o I do not teach in the classroom
Instructions - please provide a response to the following statement.
26. In addition to text, this course presented content by using other media
(for example - graphics, animation, audio, or video).
o Strongly agree
o Agree
o Disagree
o Strongly disagree
27. Developing and teaching this online course provided me with an opportunity
to reflect on how I teach in the classroom.
o Agree strongly
o Agree
o Neutral
o Disagree
o Disagree strongly
o I do not teach in the classroom
28. Think about similar courses you have developed for classroom-based delivery,
relative to those courses, how likely were you to systematically design instruction
before teaching?
o Much more systematic design of instruction online
o More systematic design of instruction online
o About the same
o Less systematic design of instruction online
o Much less systematic design of instruction online
o I do not teach in the classroom
29. I believe developing and teaching this online course has or will help me
improve the way I teach in the classroom.
o Agree strongly
o Agree
o Neutral
o Disagree
o Disagree strongly
o I do not teach in the classroom
30. Developing and teaching this online course provided me with an opportunity
to consider alternative means of assessment.
o Agree strongly
o Agree
o Neutral
o Disagree
o Disagree strongly
31. Developing and teaching this online course made me feel isolated from my
students.
o Agree strongly
o Agree
o Neutral
o Disagree
o Disagree strongly
32. Developing and teaching this online course provided me with an opportunity
to consider alternative means of instruction, i.e., new learning activities.
o Agree strongly
o Agree
o Neutral
o Disagree
o Disagree strongly
33. The course management software is very difficult to use.
o Agree strongly
o Agree
o Neutral
o Disagree
o Disagree strongly
34. It is possible to work offline in the course management software (i.e.,
to work on your course while disconnected from the internet). The ability to
work offline is very important to me.
o Agree strongly
o Agree
o Neutral
o Disagree
o Disagree strongly
What was one thing the "drove you nuts" about the course
management software:
The authors would like to express their gratitude to Dr. David Porush, Executive
Director of SUNY Learning Environments and the staff of the SUNY Learning Network
for their assistance with this research project.